Error correction of predictions from a simulation model using Random Forests
Reducing errors in predictions from forward simulation models is often achieved by model calibration or data assimilation. In our recently published manuscript, Youchen Shen and our team explore an alternative that involves correction of predictions using a machine learning algorithm (Random Forests). We use streamflow predictions from the global water balance model PCRGLOB-WB as case study. It is hypothesized that the forcings (e.g. precipitation) as well as the simulated state variables (e.g. streamflow) of the simulation are informative for the magnitude of the error in streamflow predicted by the model. In particular the use of the simulated state variables is an innovative aspect of our study.
The figure below compares the different scenarios (Basel (Rhine); NSE and KGE, larger values indicate smaller errors). Black-outlined boxes give the performance of the simulation model without error correction, for the calibrated and the uncalibrated simulation model. The coloured bars give the performance after error correction. Using only meteorogical driving variables in error correction (red bars) considerably reduces error. The use of simulated state variables (green, blue) further reduces errors.
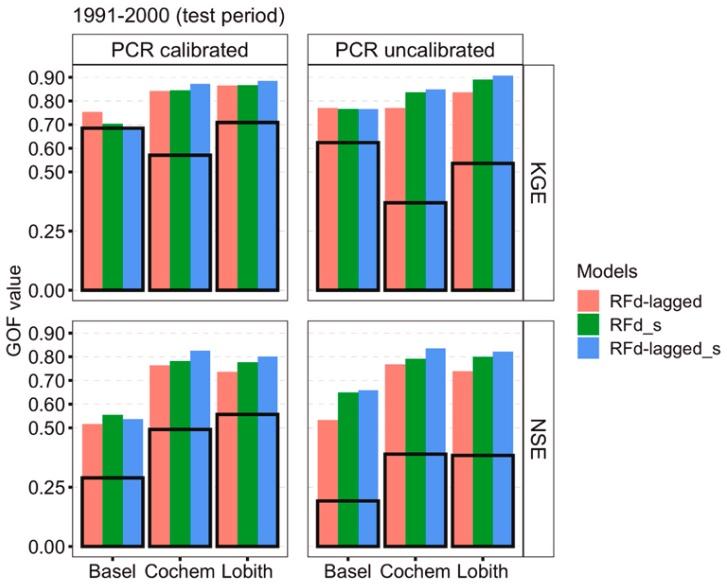
The figure below shows the effect on the predicted hydrographs (black, observed streamflow; blue calibrated simulation model; red after error correction).
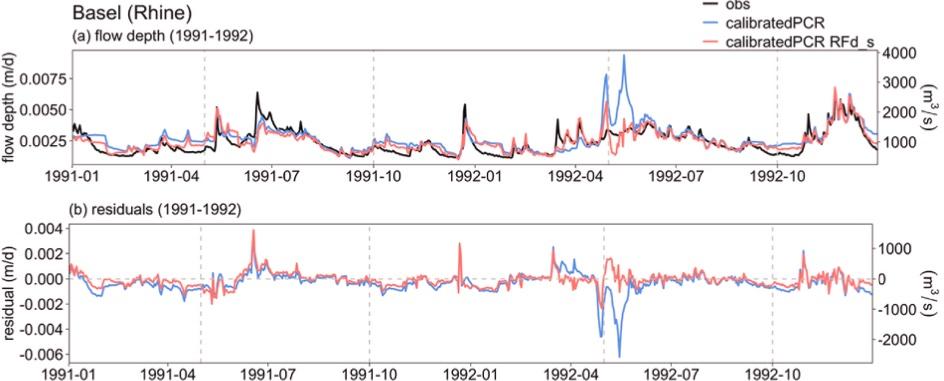
Our approach is promising as it shows that error correction using a random forest provides errors in streamflow predictions that are considerably smaller than those from a calibrated model. We also show that simulated model state is informative of the magnitude of the error. Read the full paper at https://doi.org/10.1016/j.cageo.2021.105019